Aug 9, 2023
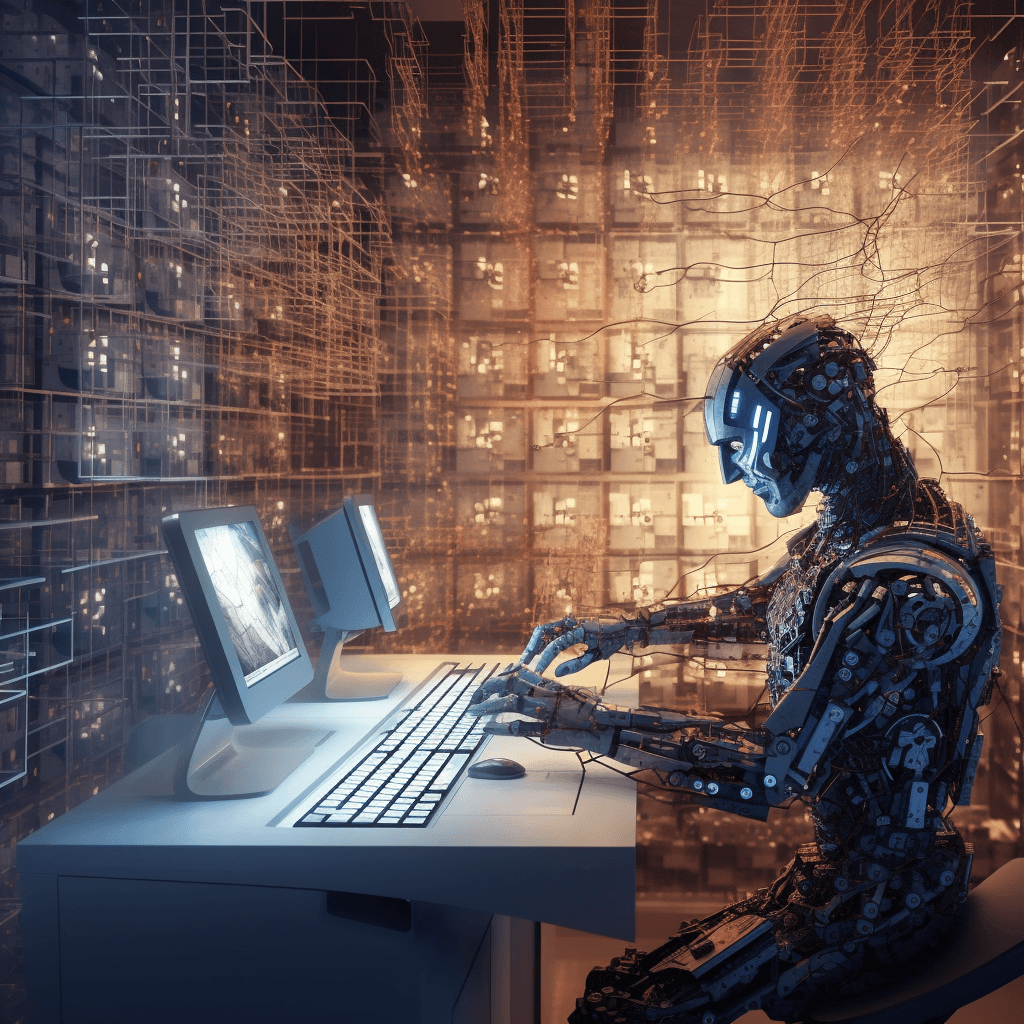
Deep Learning has emerged as a powerful tool for solving complex problems in various domains such as computer vision, natural language processing, robotics, and healthcare. With the advent of Big Data and the availability of powerful computing resources, Deep Learning has gained popularity in recent years. In this article, we will provide an overview of Deep Learning research papers, their evolution, applications, techniques, challenges, and future directions.
Evolution of Deep Learning Research
Early works in Deep Learning can be traced back to the 1940s, but it wasn't until the 1980s that significant progress was made. However, the lack of computing resources limited the scope of research. In the 2010s, the availability of Big Data and powerful GPUs led to a surge in Deep Learning research. Key researchers in this field include Geoffrey Hinton, Yann LeCun, and Yoshua Bengio.
Recent advances in Deep Learning have led to breakthroughs in various domains, including computer vision, natural language processing, and robotics. For instance, Deep Learning has been used to develop autonomous vehicles, improve healthcare outcomes, and enhance cybersecurity.
Applications of Deep Learning
Deep Learning has a wide range of applications. In computer vision, it has been used for image classification, object detection, and image segmentation. In natural language processing, Deep Learning has been used for sentiment analysis, machine translation, and speech recognition. In robotics, Deep Learning has been used for perception, control, and decision-making. In healthcare, Deep Learning has been used for disease diagnosis, drug discovery, and personalized medicine.
Deep Learning Techniques
There are several Deep Learning techniques, including Convolutional Neural Networks (CNN), Recurrent Neural Networks (RNN), Generative Adversarial Networks (GAN), and Transfer Learning. CNNs are used for image processing, while RNNs are used for sequence processing. GANs are used for generative tasks, such as image and text generation. Transfer Learning is used to transfer knowledge from one domain to another.
Challenges in Deep Learning Research
Despite its successes, Deep Learning research faces several challenges. One of the main challenges is the lack of interpretability of Deep Learning models, which limits their use in critical applications. Another challenge is the need for large amounts of data, which can be difficult to obtain in certain domains. Deep Learning models are also computationally expensive, which limits their scalability. Finally, there are ethical concerns associated with Deep Learning, such as bias and privacy issues.
Future Directions of Deep Learning Research
Future directions of Deep Learning research include Explainable AI, Federated Learning, Quantum Computing, and Integration with Other Technologies. Explainable AI aims to develop models that can provide explanations for their decisions, which is important in critical applications such as healthcare. Federated Learning aims to develop models that can be trained on decentralized data, which is important for privacy-preserving applications. Quantum Computing can enable faster computations and more powerful models, but it requires new algorithms and architectures. Integration with other technologies such as Augmented Reality, Virtual Reality, and Edge Computing can lead to new applications and use cases.
Conclusion
Deep Learning research has made significant progress in recent years and has led to breakthroughs in various domains. However, it still faces several challenges such as lack of interpretability, data scarcity, and computational complexity. Future directions of research include Explainable AI, Federated Learning, Quantum Computing, and Integration with Other Technologies.
FAQs
What is Deep Learning? Deep Learning is a subset of Machine Learning that uses artificial neural networks to learn from data and make predictions or decisions.
What are the applications of Deep Learning? Deep Learning has applications in various domains such as computer vision, natural language processing, robotics, and healthcare.
What are some key techniques used in Deep Learning? Some key techniques used in Deep Learning include Convolutional Neural Networks (CNN), Recurrent Neural Networks (RNN), Generative Adversarial Networks (GAN), and Transfer Learning.
What are some challenges in Deep Learning research? Some challenges in Deep Learning research include lack of interpretability, data scarcity, computational complexity, and ethical concerns such as bias and privacy issues.
What is the future of Deep Learning research? The future of Deep Learning research includes Explainable AI, Federated Learning, Quantum Computing, and Integration with Other Technologies.
Want to find out more about ChatGPT and other AI tools? At aicourses.com we teach you how you can thrive in the realm of marketing or improve your business with the use of Artificial Intelligence. Find more info on aicourses.com